{ "nbformat": 4, "nbformat_minor": 0, "metadata": { "colab": { "name": "Cuda_ParallelReduction.ipynb", "version": "0.3.2", "provenance": [], "collapsed_sections": [], "include_colab_link": true }, "kernelspec": { "name": "python3", "display_name": "Python 3" }, "accelerator": "GPU" }, "cells": [ { "cell_type": "markdown", "metadata": { "id": "view-in-github", "colab_type": "text" }, "source": [ "[View in Colaboratory](https://colab.research.google.com/gist/vnkdj5/11124c092c73084ce4916870856f8ba8/cuda_parallelreduction.ipynb)" ] }, { "metadata": { "id": "O4iLfcA4pArU", "colab_type": "code", "colab": { "base_uri": "https://localhost:8080/", "height": 51 }, "outputId": "a69740d4-cc37-4f59-8a6e-99561a45630e" }, "cell_type": "code", "source": [ "!pip install git+git://github.com/andreinechaev/nvcc4jupyter.git\n", "#installing nvcc jupyter extension" ], "execution_count": 15, "outputs": [ { "output_type": "stream", "text": [ "The nvcc_plugin extension is already loaded. To reload it, use:\n", " %reload_ext nvcc_plugin\n" ], "name": "stdout" } ] }, { "metadata": { "id": "Q1qw-wrEJ3q3", "colab_type": "code", "colab": { "base_uri": "https://localhost:8080/", "height": 156 }, "outputId": "c9dc310e-8365-42ec-ab3c-7e9a2f6ce0cb" }, "cell_type": "code", "source": [ "#load extension into environment\n", "%load_ext nvcc_plugin" ], "execution_count": 6, "outputs": [ { "output_type": "stream", "text": [ "Collecting git+git://github.com/andreinechaev/nvcc4jupyter.git\n", " Cloning git://github.com/andreinechaev/nvcc4jupyter.git to /tmp/pip-req-build-km0tvqr_\n", "Requirement already satisfied (use --upgrade to upgrade): NVCCPlugin==0.0.1 from git+git://github.com/andreinechaev/nvcc4jupyter.git in /usr/local/lib/python3.6/dist-packages\n", "Building wheels for collected packages: NVCCPlugin\n", " Running setup.py bdist_wheel for NVCCPlugin ... \u001b[?25l-\b \bdone\n", "\u001b[?25h Stored in directory: /tmp/pip-ephem-wheel-cache-k54f58y6/wheels/10/c2/05/ca241da37bff77d60d31a9174f988109c61ba989e4d4650516\n", "Successfully built NVCCPlugin\n" ], "name": "stdout" } ] }, { "metadata": { "id": "SM6G3Sg-m5SW", "colab_type": "text" }, "cell_type": "markdown", "source": [ "# **Problem Statement: 1.a) Implement Parallel Reduction using Min, Max, Sum and Average operations.**" ] }, { "metadata": { "id": "lIZUi6Qxp4-t", "colab_type": "code", "outputId": "c26aa332-5ac4-4e08-d7b1-55e3a1987974", "colab": { "base_uri": "https://localhost:8080/", "height": 54 } }, "cell_type": "code", "source": [ "%%cu\n", "\n", " \n", "#include<iostream>\n", "#include<cstdio>\n", "#include<cstdlib>\n", "#include<cuda_runtime.h>\n", "using namespace std;\n", "\n", "\n", "__global__ void minimum(int *input)\n", "{\n", "\tint tid=threadIdx.x;\n", "\tauto step_size=1;\n", " int number_of_threads=blockDim.x;\n", " \n", " while(number_of_threads>0)\n", " {\n", " if(tid<number_of_threads)\n", " {\n", " int first=tid*step_size*2;\n", " int second=first+step_size;\n", " if(input[second]<input[first])\n", " input[first]=input[second];\n", " }\n", " step_size=step_size*2;\n", " number_of_threads/=2;\n", " }\n", "\n", "}\n", "\n", "__global__ void max(int *input)\n", "{\n", " int tid=threadIdx.x;\n", " auto step_size=1;\n", " int number_of_threads=blockDim.x;\n", " \n", " while(number_of_threads>0)\n", " {\n", " if(tid<number_of_threads)\n", " {\n", " int first=tid*step_size*2;\n", " int second=first+step_size;\n", " if(input[second]>input[first])\n", " input[first]=input[second];\n", " }\n", " step_size*=2;\n", " number_of_threads/=2;\n", " }\n", "}\n", "\n", "__global__ void sum(int *input)\n", "{\n", " const int tid=threadIdx.x;\n", " auto step_size=1;\n", " int number_of_threads=blockDim.x;\n", " while(number_of_threads>0)\n", " {\n", " if(tid<number_of_threads)\n", " {\n", " const int first=tid*step_size*2;\n", " const int second=first+step_size;\n", " input[first]=input[first]+input[second];\n", " }\n", " step_size = step_size*2;; \n", "\t\tnumber_of_threads =number_of_threads/2;\n", " }\n", "}\n", "\n", "__global__ void average(int *input) //You can use above sum() to calculate sum and divide it by num_of_elememts\n", "{\n", " const int tid=threadIdx.x;\n", " auto step_size=1;\n", " int number_of_threads=blockDim.x;\n", " int totalElements=number_of_threads*2;\n", " while(number_of_threads>0)\n", " {\n", " if(tid<number_of_threads)\n", " {\n", " const int first=tid*step_size*2;\n", " const int second=first+step_size;\n", " input[first]=input[first]+input[second];\n", " }\n", " step_size = step_size*2;; \n", "\t\tnumber_of_threads =number_of_threads/2;\n", " }\n", " input[0]=input[0]/totalElements;\n", "}\n", "\n", "int main()\n", "{\n", "\n", "\tcout<<\"Enter the no of elements\"<<endl;\n", "\tint n;\n", "\tn=10;\n", " srand(n);\n", "\tint *arr=new int[n];\n", " int min=20000;\n", " //# Generate Input array using rand()\n", "\tfor(int i=0;i<n;i++)\n", "\t{\n", "\t\tarr[i]=rand()%20000;\n", " if(arr[i]<min)\n", " min=arr[i];\n", " cout<<arr[i]<<\" \";\n", "\t}\n", "\n", "\tint size=n*sizeof(int); //calculate no. of bytes for array\n", "\tint *arr_d,result1;\n", "\t\n", " //# Allocate memory for min Operation\n", "\tcudaMalloc(&arr_d,size);\n", "\tcudaMemcpy(arr_d,arr,size,cudaMemcpyHostToDevice);\n", "\n", " minimum<<<1,n/2>>>(arr_d);\n", "\n", "\tcudaMemcpy(&result1,arr_d,sizeof(int),cudaMemcpyDeviceToHost);\n", "\n", "\tcout<<\"The minimum element is \\n \"<<result1<<endl;\n", " \n", " cout<<\"The min element (using CPU) is\"<<min;\n", " \n", " \n", " //#MAX OPERATION \n", " int *arr_max,maxValue;\n", " cudaMalloc(&arr_max,size);\n", "\tcudaMemcpy(arr_max,arr,size,cudaMemcpyHostToDevice);\n", "\n", " max<<<1,n/2>>>(arr_max);\n", "\n", "\tcudaMemcpy(&maxValue,arr_max,sizeof(int),cudaMemcpyDeviceToHost);\n", "\n", "\tcout<<\"The maximum element is \\n \"<<maxValue<<endl;\n", " \n", " //#SUM OPERATION \n", " int *arr_sum,sumValue;\n", " cudaMalloc(&arr_sum,size);\n", "\tcudaMemcpy(arr_sum,arr,size,cudaMemcpyHostToDevice);\n", "\n", " sum<<<1,n/2>>>(arr_sum);\n", "\n", "\tcudaMemcpy(&sumValue,arr_sum,sizeof(int),cudaMemcpyDeviceToHost);\n", "\n", "\tcout<<\"The sum of elements is \\n \"<<sumValue<<endl; \n", " \n", " cout<<\"The average of elements is \\n \"<<(sumValue/n)<<endl; \n", " \n", " //# OR-----------\n", " \n", " //#AVG OPERATION \n", " int *arr_avg,avgValue;\n", " cudaMalloc(&arr_avg,size);\n", "\tcudaMemcpy(arr_avg,arr,size,cudaMemcpyHostToDevice);\n", "\n", " average<<<1,n/2>>>(arr_avg);\n", "\n", "\tcudaMemcpy(&avgValue,arr_avg,sizeof(int),cudaMemcpyDeviceToHost);\n", "\n", "\tcout<<\"The average of elements is \\n \"<<avgValue<<endl; \n", " \n", " \n", " //# Free all allcated device memeory\n", " cudaFree(arr_d);\n", " cudaFree(arr_sum);\n", " cudaFree(arr_max);\n", " cudaFree(arr_avg);\n", " \n", " \n", " \n", "\n", "return 0;\n", "\n", "}" ], "execution_count": 49, "outputs": [ { "output_type": "execute_result", "data": { "text/plain": [ "'Enter the no of elements\\n9295 2008 8678 8725 418 2377 12675 13271 4747 2307 The minimum element is \\n 418\\nThe min element (using CPU) is418The maximum element is \\n 13271\\nThe sum of elements is \\n 57447\\nThe average of elements is \\n 5744\\nThe average of elements is \\n 5744\\n'" ] }, "metadata": { "tags": [] }, "execution_count": 49 } ] }, { "metadata": { "id": "2rPE4qXilqBb", "colab_type": "text" }, "cell_type": "markdown", "source": [ "**Problem Statement: 1.a) Implement Parallel Reduction using Min, Max, Sum and Average operations.**\n", "\n", "Reference: [Parallel Reduction in CUDA Explained](http://www.elemarjr.com/en/2018/03/parallel-reduction-in-cuda/)\n", "\n", "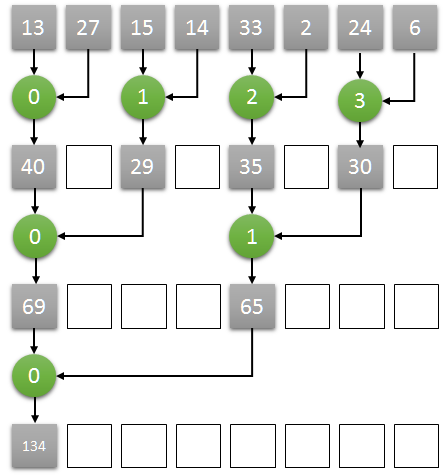\n", "\n", "Here is the main idea:\n", "\n", "1. Assuming N as the number of the elements in an array, we start N/2 threads, one thread for each two elements\n", "2. Each thread computes the sum of the corresponding two elements, storing the result at the position of the first one.\n", "3. Iteratively, each step:\n", " the number of threads halved (for example, starting with 4, then 2, then 1)\n", " doubles the step size between the corresponding two elements (starting with 1, then 2, then 4)\n", "4. after some iterations, the reduction result will be stored in the first element of the array." ] }, { "metadata": { "id": "jfDtsKNjpAm2", "colab_type": "code", "colab": {} }, "cell_type": "code", "source": [ "" ], "execution_count": 0, "outputs": [] }, { "metadata": { "id": "LlPOyb2oonL1", "colab_type": "code", "colab": {} }, "cell_type": "code", "source": [ "" ], "execution_count": 0, "outputs": [] } ] }