Last active
May 20, 2024 16:39
-
-
Save blaylockbk/8b469f2c79660ebdd18915202e0802a6 to your computer and use it in GitHub Desktop.
Template for Python multiprocessing and multithreading
This file contains bidirectional Unicode text that may be interpreted or compiled differently than what appears below. To review, open the file in an editor that reveals hidden Unicode characters.
Learn more about bidirectional Unicode characters
import multiprocessing | |
from multiprocessing.dummy import Pool as ThreadPool | |
import numpy as np | |
def my_multipro(items, func, max_cpus=12): | |
"""Do an embarrassingly parallel task using multiprocessing. | |
Use this for CPU bound tasks. | |
Parameters | |
---------- | |
items : list | |
Items to be acted on. | |
func : function | |
Function to apply to each item. | |
max_cpus : int | |
Limit the number of CPUs to use | |
""" | |
# Don't use more CPUs than you have or more than there are items to process | |
cpus = np.min([max_cpus, multiprocessing.cpu_count(), len(items)]) | |
print(f"Using {cpus} cpus to process {len(items)} chunks.") | |
print("".join(["🔳" for i in items])) | |
with multiprocessing.Pool(cpus) as p: | |
results = p.map(func, items) | |
p.close() | |
p.join() | |
print("Finished!") | |
return results | |
def my_multithread(items, func, max_threads=12): | |
"""Do an embarrassingly parallel task using multithreading. | |
Use this for IO bound tasks. | |
Parameters | |
---------- | |
items : list | |
Items to be acted on. | |
func : function | |
Function to apply to each item. | |
max_cpus : int | |
Limit the number of CPUs to use | |
""" | |
# Don't use more threads than there are items to process | |
threads = np.min([max_threads, len(items)]) | |
print(f"Using {threads} threads to process {len(items)} items.") | |
print("".join(["🔳" for i in items])) | |
with ThreadPool(threads) as p: | |
results = p.map(func, items) | |
p.close() | |
p.join() | |
print("Finished!") | |
return results | |
def do_this(i): | |
"""This function will be applied to every item given.""" | |
r = np.mean(i) | |
print("✅", end="") | |
return r | |
items = [ | |
[1, 2, 3, 4], | |
[2, 3, 4, 5], | |
[3, 4, 5, 6], | |
[4, 5, 6, 7], | |
[5, 6, 7, 8], | |
[6, 7, 8, 9], | |
] | |
a = my_multipro(items, do_this) | |
b = my_multithread(items, do_this) |
Is there a way to get max number of threads in multithreading that a "system" can support like cpu_count
for multiprocessing.
from multiprocessing.dummy import Pool as ThreadPool
threads = 4
with ThreadPool(threads) as p:
results = p.map(print, list(range(10)))
p.close()
p.join()
So instead of 4 I can let the script determine it. I do not know how the underlying system works here so the question might be naive.
@urwa I would look at this for that answer: https://stackoverflow.com/questions/48970857/maximum-limit-on-number-of-threads-in-python
Sign up for free
to join this conversation on GitHub.
Already have an account?
Sign in to comment
Multipro Helper
This function helps sends a list of jobs to either basic multiprocessing or multithreading, or sequential list comprehension.
And this will make a plot of performance for a number of different pool sizes. It can help you see where you're getting diminishing returns for a larger number of Pools, and help you see if your problem is a CPU bound or IO bound process. Use multiprocessing for CPU-bound process and multithreading for IO-bound process.
For example, in this example of reading a file and processing the data in it, this is a CPU-bound process and should use multiprocessing to spead it up, but you don't get much speed up if you use more than 5 or 6 CPUs.
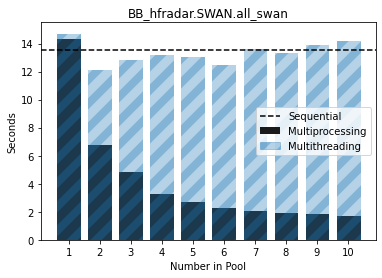