Last active
September 2, 2021 07:59
-
-
Save crypt3lx2k/cec6ad66b948fe0e77a7b1e6d2205bf4 to your computer and use it in GitHub Desktop.
Training model in tensorflow for tflite with 8-bit integer quantization
This file contains bidirectional Unicode text that may be interpreted or compiled differently than what appears below. To review, open the file in an editor that reveals hidden Unicode characters.
Learn more about bidirectional Unicode characters
#! /usr/bin/env python | |
import tensorflow as tf | |
def inference_fn(x, training=False): | |
net = x | |
net = tf.layers.flatten(net) | |
net = tf.layers.dense(net, 512, activation=tf.nn.relu) | |
net = tf.layers.dropout(net, 0.2, training=training) | |
logits = tf.layers.dense(net, 10, activation=None) | |
probs = tf.nn.softmax(logits) | |
return dict(logits=logits, probs=probs) | |
def model_fn(x, y=None, training=False, quantize=False, **params): | |
global_step = tf.train.create_global_step() | |
inference_model = inference_fn(x, training=training) | |
if not training: | |
if quantize: | |
tf.contrib.quantize.create_eval_graph() | |
return inference_model | |
loss = tf.losses.sparse_softmax_cross_entropy( | |
labels=y, logits=inference_model['logits'] | |
) | |
global_variables = tf.global_variables() | |
if quantize: | |
tf.contrib.quantize.create_training_graph( | |
quant_delay=params.get('quant_delay', 0) | |
) | |
opt = tf.train.AdamOptimizer(params['learning_rate']) | |
train_op = opt.minimize(loss, global_step=global_step) | |
inference_model['global_variables'] = global_variables | |
inference_model['loss'] = loss | |
inference_model['train_op'] = train_op | |
return inference_model |
This file contains bidirectional Unicode text that may be interpreted or compiled differently than what appears below. To review, open the file in an editor that reveals hidden Unicode characters.
Learn more about bidirectional Unicode characters
#! /usr/bin/env python | |
import model | |
import tensorflow as tf | |
import os | |
def run_epoch(x, y, session, model_train, data, train_size, batch_size): | |
x_train, y_train = data | |
total_loss = 0.0 | |
for i in range(0, train_size, batch_size): | |
loss, _ = session.run( | |
[ | |
model_train['loss'], | |
model_train['train_op'] | |
], | |
feed_dict={ | |
x : x_train[i:i+batch_size], | |
y : y_train[i:i+batch_size] | |
} | |
) | |
total_loss += loss | |
return total_loss / (train_size//batch_size) | |
def main(): | |
batch_size = 32 | |
num_epochs = 5 | |
mnist = tf.keras.datasets.mnist | |
(x_train, y_train),(x_test, y_test) = mnist.load_data() | |
x_train, x_test = x_train / 255.0, x_test / 255.0 | |
train_size = x_train.shape[0] | |
test_size = x_test.shape[0] | |
graph = tf.Graph() | |
with graph.as_default(): | |
with tf.variable_scope('inputs'): | |
x = tf.placeholder(dtype=tf.float32, shape=[None, 28, 28]) | |
y = tf.placeholder(dtype=tf.int32, shape=[None]) | |
with tf.variable_scope('model'): | |
model_train = model.model_fn( | |
x, y, training=True, learning_rate=1e-3 | |
) | |
init_op = tf.global_variables_initializer() | |
saver = tf.train.Saver(sharded=True) | |
graph.finalize() | |
with tf.Session(graph=graph) as session: | |
session.run(init_op) | |
for e in range(num_epochs): | |
loss = run_epoch(x, y, session, model_train, (x_train, y_train), train_size, batch_size) | |
print('epoch {} : {}'.format(e, loss)) | |
if not os.path.exists('checkpoints/'): | |
os.makedirs('checkpoints/') | |
saver.save( | |
session, 'checkpoints/model.ckpt', | |
global_step=tf.train.get_global_step() | |
) | |
return 0 | |
if __name__ == '__main__': | |
exit(main()) |
This file contains bidirectional Unicode text that may be interpreted or compiled differently than what appears below. To review, open the file in an editor that reveals hidden Unicode characters.
Learn more about bidirectional Unicode characters
#! /usr/bin/env python | |
import model | |
import tensorflow as tf | |
def run_epoch(x, y, session, model_train, data, train_size, batch_size): | |
x_train, y_train = data | |
total_loss = 0.0 | |
for i in range(0, train_size, batch_size): | |
loss, _ = session.run( | |
[ | |
model_train['loss'], | |
model_train['train_op'] | |
], | |
feed_dict={ | |
x : x_train[i:i+batch_size], | |
y : y_train[i:i+batch_size] | |
} | |
) | |
total_loss += loss | |
return total_loss / (train_size//batch_size) | |
def main(): | |
batch_size = 32 | |
num_epochs = 1 | |
mnist = tf.keras.datasets.mnist | |
(x_train, y_train),(x_test, y_test) = mnist.load_data() | |
x_train, x_test = x_train / 255.0, x_test / 255.0 | |
train_size = x_train.shape[0] | |
test_size = x_test.shape[0] | |
graph = tf.Graph() | |
with graph.as_default(): | |
with tf.variable_scope('inputs'): | |
x = tf.placeholder(dtype=tf.float32, shape=[None, 28, 28]) | |
y = tf.placeholder(dtype=tf.int32, shape=[None]) | |
with tf.variable_scope('model'): | |
model_train = model.model_fn( | |
x, y, training=True, learning_rate=1e-3, quantize=True | |
) | |
init_op = tf.global_variables_initializer() | |
restorer = tf.train.Saver(model_train['global_variables'], sharded=True) | |
saver = tf.train.Saver(sharded=True) | |
graph.finalize() | |
with tf.Session(graph=graph) as session: | |
checkpoint = tf.train.latest_checkpoint('checkpoints/') | |
session.run(init_op) | |
restorer.restore(session, checkpoint) | |
for e in range(num_epochs): | |
loss = run_epoch(x, y, session, model_train, (x_train, y_train), train_size, batch_size) | |
print('epoch {} : {}'.format(e, loss)) | |
saver.save( | |
session, 'checkpoints/model.ckpt', | |
global_step=tf.train.get_global_step() | |
) | |
return 0 | |
if __name__ == '__main__': | |
exit(main()) |
This file contains bidirectional Unicode text that may be interpreted or compiled differently than what appears below. To review, open the file in an editor that reveals hidden Unicode characters.
Learn more about bidirectional Unicode characters
#! /usr/bin/env python | |
import model | |
import numpy as np | |
import tensorflow as tf | |
def main(): | |
graph = tf.Graph() | |
with graph.as_default(): | |
with tf.variable_scope('inputs'): | |
x = tf.placeholder(dtype=tf.float32, shape=[None, 28, 28]) | |
with tf.variable_scope('model'): | |
model_infer = model.model_fn(x, quantize=True) | |
saver = tf.train.Saver(sharded=True) | |
graph.finalize() | |
with tf.Session(graph=graph) as session: | |
checkpoint = tf.train.latest_checkpoint('checkpoints/') | |
saver.restore(session, checkpoint) | |
builder = tf.saved_model.Builder('exports') | |
signature_def = tf.saved_model.predict_signature_def( | |
inputs={'x' : x}, | |
outputs=model_infer | |
) | |
builder.add_meta_graph_and_variables( | |
sess=session, | |
tags=[ | |
tf.saved_model.tag_constants.SERVING | |
], | |
signature_def_map={ | |
tf.saved_model.signature_constants.DEFAULT_SERVING_SIGNATURE_DEF_KEY : signature_def | |
}, | |
saver=saver | |
) | |
builder.save() | |
return 0 | |
if __name__ == '__main__': | |
exit(main()) |
This file contains bidirectional Unicode text that may be interpreted or compiled differently than what appears below. To review, open the file in an editor that reveals hidden Unicode characters.
Learn more about bidirectional Unicode characters
#! /usr/bin/env python | |
import tensorflow.contrib.lite as lite | |
import os | |
converter = lite.TFLiteConverter.from_saved_model('exports') | |
converter.inference_type = lite.constants.QUANTIZED_UINT8 | |
converter.inference_input_type = lite.constants.QUANTIZED_UINT8 | |
converter.quantized_input_stats = {'inputs/Placeholder' : (0.0, 255.0)} | |
if not os.path.exists('graphviz/'): | |
os.makedirs('graphviz/') | |
converter.dump_graphviz_dir = 'graphviz' | |
flatbuffer = converter.convert() | |
with open('mnist.tflite', 'wb') as outfile: | |
outfile.write(flatbuffer) |
This file contains bidirectional Unicode text that may be interpreted or compiled differently than what appears below. To review, open the file in an editor that reveals hidden Unicode characters.
Learn more about bidirectional Unicode characters
#! /usr/bin/env python | |
import numpy as np | |
import tensorflow as tf | |
import tensorflow.contrib.lite as lite | |
mnist = tf.keras.datasets.mnist | |
batch_size = 32 | |
_,(x_test, y_test) = mnist.load_data() | |
interpreter = lite.Interpreter('mnist.tflite') | |
input_info = interpreter.get_input_details()[0] | |
output_info = interpreter.get_output_details()[0] | |
interpreter.resize_tensor_input(input_info['index'], (batch_size, 28, 28)) | |
interpreter.allocate_tensors() | |
interpreter.set_tensor(input_info['index'], x_test[0:batch_size]) | |
interpreter.invoke() | |
probs = interpreter.get_tensor(output_info['index']) | |
print('predicted={}, label={}'.format(np.argmax(probs, axis=-1), y_test[0:batch_size])) |
Sign up for free
to join this conversation on GitHub.
Already have an account?
Sign in to comment
Resulting tflite flatbuffer graph
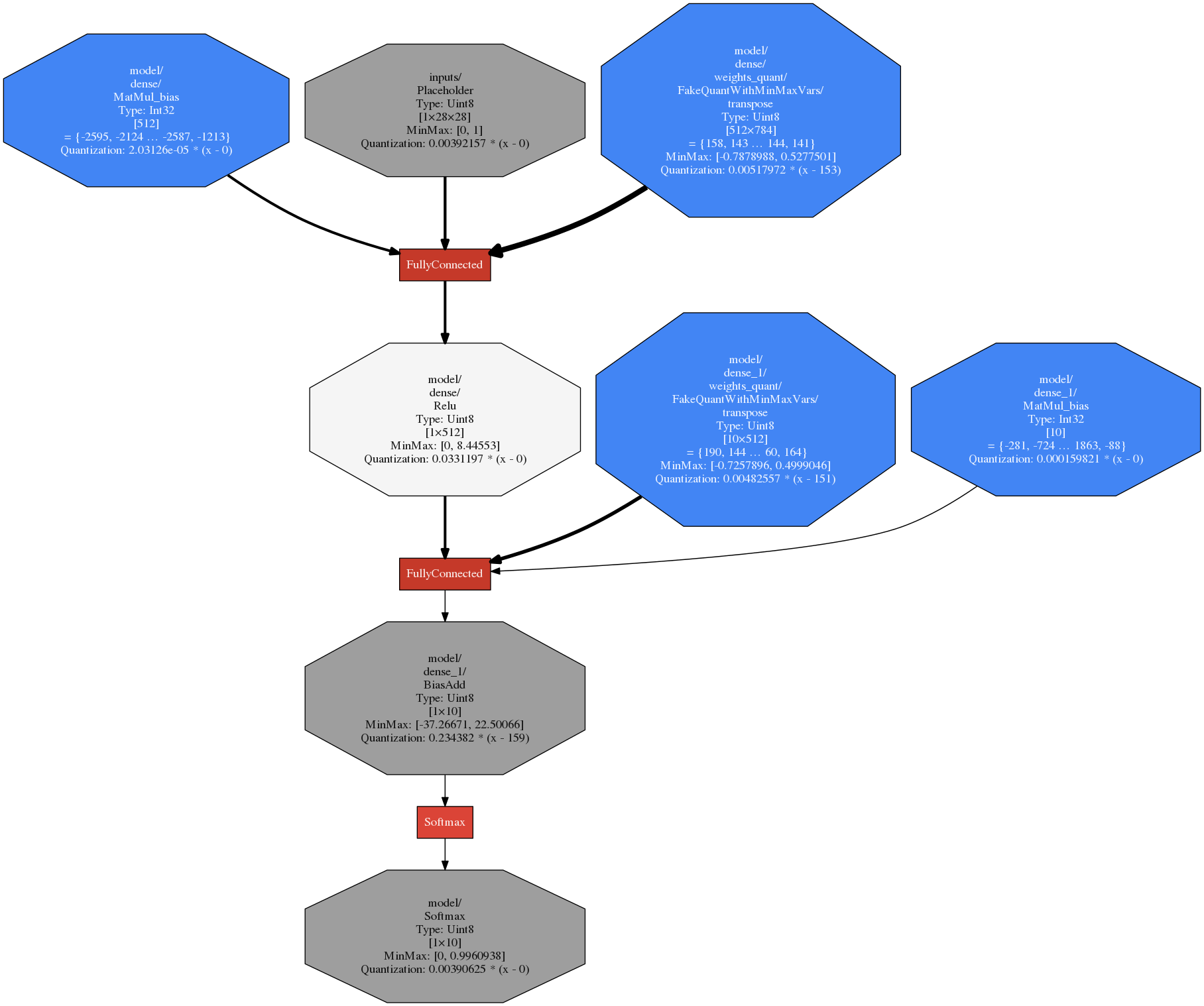