Last active
October 24, 2023 03:49
-
-
Save kohiro37/d8dd770421e89fbf2c2907d4400931f0 to your computer and use it in GitHub Desktop.
夏目漱石の『こころ』からインタラクティブな共起ネットワークを作成
This file contains bidirectional Unicode text that may be interpreted or compiled differently than what appears below. To review, open the file in an editor that reveals hidden Unicode characters.
Learn more about bidirectional Unicode characters
""" 夏目漱石の『こころ』からインタラクティブな共起ネットワークを作成 | |
コードの説明は以下のブログ | |
Pythonでインタラクティブな共起ネットワークを作成する | |
https://irukanobox.blogspot.com/2021/02/python.html | |
【テキストデータ】 | |
青空文庫の夏目漱石の『こころ』(773_ruby_5968.zip)を解凍したファイル(kokoro.txt) | |
https://www.aozora.gr.jp/cards/000148/card773.html | |
【動作環境】 | |
-Ubuntu 20.04 | |
-Python 3.8.5 | |
-Jupyter Lab | |
-Node.js 14.10.0 | |
-MeCab 0.996 | |
https://taku910.github.io/mecab/ | |
-Graphviz 2.43.0 | |
https://www.graphviz.org/ | |
Jupyter Lab拡張機能 | |
-jupyterlab-plotly | |
動作時のPythonライブラリ | |
-mecab-python3 1.0.3 | |
-networkx 2.5 | |
-numpy 1.19.5 | |
-plotly 4.14.3 | |
-pygraphviz 1.7 | |
-scikit-learn 0.24.1 | |
-scipy 1.6.0 | |
""" | |
import re | |
from itertools import combinations | |
from collections import Counter | |
import MeCab | |
import numpy as np | |
from sklearn.feature_extraction.text import CountVectorizer | |
from sklearn.metrics import pairwise_distances | |
from scipy.spatial.distance import pdist | |
import networkx as nx | |
from networkx.drawing import nx_agraph | |
from plotly import graph_objs as go | |
# 対象の品詞 | |
TARGET_POS1 = ['名詞'] | |
# 対象の詳細分類1 | |
TARGET_POS2 = ['サ変接続', 'ナイ形容詞語幹', '形容動詞語幹', '一般', '固有名詞'] | |
# ストップワード | |
STOP_WORDS = ['*'] | |
def ruby2txt(ruby): | |
#ルビなどの作品本文以外の文字や記号を取り除く | |
# テキスト上部の【テキスト中に現れる記号について】箇所の除去 | |
txt = re.split(r'-{50,}', ruby)[2] | |
# テキスト下部の「底本:~」の除去 | |
txt = re.split(r'底本:', txt)[0] | |
# ルビ、ルビの付く文字列の始まりを特定する記号、入力者注を除去 | |
txt = re.sub(r'《.*?》|[#.*?]||', '', txt) | |
# テキスト前後の空白を除去 | |
return txt.strip() | |
def remove_blank(chapter): | |
# 空白行と段落先頭の空白を削除 | |
lines = chapter.splitlines() | |
# 空白行削除 | |
# 行頭の空白削除 | |
lines_cleaned = [l.strip() for l in lines if len(l) != 0] | |
return '\n'.join(lines_cleaned) | |
def doc2chapter(doc): | |
# 文章を章ごとに分割 | |
# タイトル削除 | |
doc = doc.replace('上 先生と私', '').replace('中 両親と私', '').replace('下 先生と遺書', '') | |
# 章番号で章ごとに分割 | |
doc_split = re.split('[一二三四五六七八九十]{1,3}\n', doc) | |
# 先頭は空白行なので削除 | |
del doc_split[0] | |
print('Total chapter number: ', len(doc_split)) | |
chapter_l = list(map(remove_blank, doc_split)) | |
return chapter_l | |
def chapter2bform(chapter_l): | |
# 章ごとに形態素解析して単語の原型のリストを作成 | |
m = MeCab.Tagger('-Ochasen') | |
m.parse('') | |
bform_2l = [] | |
for i, chapter in enumerate(chapter_l): | |
node = m.parseToNode(chapter) | |
bform_l = [] | |
while node: | |
feature_split = node.feature.split(',') | |
pos1 = feature_split[0] | |
pos2 = feature_split[1] | |
base_form = feature_split[6] | |
if pos1 in TARGET_POS1 and pos2 in TARGET_POS2 and base_form not in STOP_WORDS: | |
bform_l.append(base_form) | |
node = node.next | |
bform_2l.append(bform_l) | |
print('Term number of chapter {}: '.format(i+1), len(bform_l)) | |
return bform_2l | |
def filter_word_by_freqency(texts, freq_top=80): | |
# Document frequency | |
c_word = Counter([word for t in texts for word in set(t)]) | |
top_word = [word for word, cnt in c_word.most_common(freq_top)] | |
texts_fitered = [] | |
for t in texts: | |
filtered = list(set(top_word).intersection(set(t))) | |
if len(filtered) > 0: | |
texts_fitered.append(filtered) | |
return texts_fitered | |
def compute_jaccard_coef(texts, edge_top): | |
# 対象をDocument Frequency上位に限定 | |
#texts_filtered = filter_word_by_freqency(texts, top = freq_top) | |
# 単語リストをタブ区切りテキストに変換 | |
tab_separated = ['\t'.join(t) for t in texts] | |
# tokenizerでタブで分割するように指定 | |
vectorizer = CountVectorizer(lowercase=False, tokenizer=lambda x: x.split('\t')) | |
vec = vectorizer.fit_transform(tab_separated) | |
# 単語リスト | |
words = vectorizer.get_feature_names() | |
# 0/1のベクトルにするためにカウント1以上はすべて1にする | |
vec_one = (vec.toarray() >= 1).astype(int).transpose() | |
# pdistを使うと結果は密行列(condensed matrix)で得られる | |
# pdistで得られるのは距離なので、係数にするために1から引く | |
jaccard_coef = 1 - pdist(vec_one, metric='jaccard') | |
# 密行列の順番はitertools.combinationsnの結果と一致するのでcombinationsでJaccard係数に対応する単語ペアを作成する | |
# How does condensed distance matrix work? (pdist) | |
# https://stackoverflow.com/questions/13079563/how-does-condensed-distance-matrix-work-pdist | |
w_pair = list(combinations(words, 2)) | |
# 単語ペアをキーとするJaccard係数のdict | |
dict_jaccard = {pair: value for pair, value in zip(w_pair, jaccard_coef)} | |
# Jaccard係数はネットワーク図のedgeに相当する | |
# その数を一定数に限定する | |
dict_jaccard = dict(sorted(dict_jaccard.items(), key = lambda x: x[1], reverse = True)[:edge_top]) | |
return dict_jaccard | |
def build_coonw(texts, freq_top=80, edge_top=60): | |
# 対象をDocument Frequency上位に限定 | |
texts_filtered = filter_word_by_freqency(texts, freq_top) | |
dict_jaccard = compute_jaccard_coef(texts_filtered, edge_top) | |
print('dict_jaccard=', dict_jaccard) | |
# Document frequency | |
df = Counter([word for t in texts_filtered for word in set(t)]) | |
# nodeリスト | |
nodes = sorted(set([word for pair in dict_jaccard.keys() for word in pair])) | |
# 単語出現数でnodeサイズを変更する | |
c_word = {n: df[n] for n in nodes} | |
G = nx.Graph() | |
# 接点/単語(node)の追加 | |
G.add_nodes_from(nodes) | |
print('Number of nodes: {}'.format(G.number_of_nodes())) | |
# 線(edge)の追加 | |
for pair, coef in dict_jaccard.items(): | |
G.add_edge(pair[0], pair[1], weight=coef) | |
print('Number of edges: {}'.format(G.number_of_edges())) | |
# nodeの配置方法の指定 | |
seed = 0 | |
np.random.seed(seed) | |
pos = nx_agraph.graphviz_layout( | |
G, | |
prog='neato', | |
args='-Goverlap="scalexy" -Gsep="+6" -Gnodesep=0.8 -Gsplines="polyline" -GpackMode="graph" -Gstart={}'.format(seed)) | |
# nodeの色をページランクアルゴリズムによる重要度により変える | |
pr = nx.pagerank(G) | |
# インタラクティブな共起ネットワークの可視化 | |
build_interactive_network(G, pos, list(c_word.values()), list(pr.values())) | |
def build_interactive_network(G, pos, node_sizes, node_colors): | |
# edgeデータの作成 | |
edge_x = [] | |
edge_y = [] | |
for edge in G.edges(): | |
x0, y0 = pos[edge[0]] | |
x1, y1 = pos[edge[1]] | |
edge_x.append(x0) | |
edge_x.append(x1) | |
edge_x.append(None) | |
edge_y.append(y0) | |
edge_y.append(y1) | |
edge_y.append(None) | |
edge_trace = go.Scatter( | |
x=edge_x, y=edge_y, | |
line=dict(width=0.5, color='#888'), | |
hoverinfo='none', | |
mode='lines') | |
# nodeデータの作成 | |
node_x = [] | |
node_y = [] | |
for node in G.nodes(): | |
x, y = pos[node] | |
node_x.append(x) | |
node_y.append(y) | |
# nodeの色、サイズ、マウスオーバーしたときに表示するテキストの設定 | |
node_trace = go.Scatter( | |
x=node_x, | |
y=node_y, | |
text=list(G.nodes()), | |
hovertext=node_sizes, | |
textposition='top center', | |
mode='markers+text', | |
hoverinfo='text', | |
marker=dict( | |
showscale=True, | |
colorscale='Portland', | |
reversescale=False, | |
color=node_colors, | |
size=node_sizes, | |
colorbar=dict( | |
thickness=15, | |
title='Page Ranking', | |
), | |
line_width=2)) | |
data = [edge_trace, node_trace] | |
# レイアウトの設定 | |
layout=go.Layout( | |
paper_bgcolor='rgba(0,0,0,0)', | |
plot_bgcolor='rgba(0,0,0,0)', | |
showlegend=False, | |
hovermode='closest', | |
margin=dict(b=10, l=5, r=5, t=10), | |
font=dict(size=10), | |
xaxis=dict(showgrid=False, zeroline=False, showticklabels=False), | |
yaxis = dict(showgrid = False, zeroline = False, showticklabels = False)) | |
fig = go.Figure(data=data, layout=layout) | |
fig.show() | |
# 青空文庫の『こころ』テキストの読み込み | |
with open('kokoro.txt', mode='r', encoding='shift-jis') as f: | |
doc = f.read() | |
# ルビなどの除去 | |
text = ruby2txt(doc) | |
# 章ごとの単語原型リスト | |
bform_2l = chapter2bform(doc2chapter(text)) | |
# インタラクティブ共起ネットワークの作成 | |
build_coonw(bform_2l, freq_top=80, edge_top=60) |
Sign up for free
to join this conversation on GitHub.
Already have an account?
Sign in to comment
作成された共起ネットワーク。
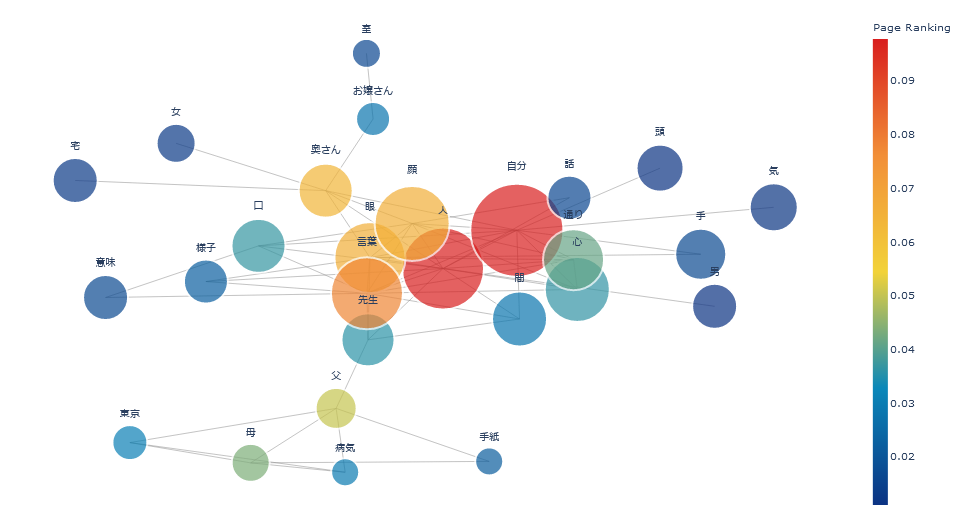